List of metaphor-based metaheuristics
This is a chronologically ordered list of metaphor-based metaheuristics and swarm intelligence algorithms.
Algorithms
Simulated annealing (Kirkpatrick et al. 1983)
Simulated annealing (SA) is a probabilistic technique inspired by a heat treatment method in metallurgy. It is often used when the search space is discrete (e.g., all tours that visit a given set of cities). For problems where finding the precise global optimum is less important than finding an acceptable local optimum in a fixed amount of time, simulated annealing may be preferable to alternatives such as gradient descent.
Simulated annealing interprets slow cooling as a slow decrease in the probability of accepting worse solutions as it explores the solution space. Accepting worse solutions is a fundamental property of metaheuristics because it allows for a more extensive search for the optimal solution.
Ant colony optimization (Dorigo, 1992)
The ant colony optimization algorithm (ACO) is a probabilistic technique for solving computational problems which can be reduced to finding good paths through graphs. Initially proposed by Marco Dorigo in 1992 in his PhD thesis,[1][2] the first algorithm was aiming to search for an optimal path in a graph, based on the behavior of ants seeking a path between their colony and a source of food. The original idea has since diversified to solve a wider class of numerical problems, and as a result, several problems have emerged, drawing on various aspects of the behavior of ants. From a broader perspective, ACO performs a model-based search[3] and shares some similarities with Estimation of Distribution Algorithms.
Particle swarm optimization (Kennedy & Eberhart 1995)
Particle swarm optimization (PSO) is a computational method that optimizes a problem by iteratively trying to improve a candidate solution with regard to a given measure of quality. It solves a problem by having a population of candidate solutions, here dubbed particles, and moving these particles around in the search-space according to simple mathematical formulae over the particle's position and velocity. Each particle's movement is influenced by its local best known position, but is also guided toward the best known positions in the search-space, which are updated as better positions are found by other particles. This is expected to move the swarm toward the best solutions.
PSO is originally attributed to Kennedy, Eberhart and Shi[4][5] and was first intended for simulating social behaviour,[6] as a stylized representation of the movement of organisms in a bird flock or fish school. The algorithm was simplified and it was observed to be performing optimization. The book by Kennedy and Eberhart[7] describes many philosophical aspects of PSO and swarm intelligence. An extensive survey of PSO applications is made by Poli.[8][9] Recently, a comprehensive review on theoretical and experimental works on PSO has been published by Bonyadi and Michalewicz.[10]
Harmony search (Geem, Kim & Loganathan 2001)
Harmony search is a phenomenon-mimicking metaheuristic introduced in 2001 by Zong Woo Geem, Joong Hoon Kim, and G. V. Loganathan.[11] Harmony search is inspired by the improvisation process of jazz musicians. Harmony search has been strongly criticized for being a special case of the well-established Evolution Strategies algorithm.[12] However the rebuttal provided in [13] gives ample evidence that HS is not a special case of Evolutionary strategies.
The Harmony search (HS) is a relatively simple yet very efficient evolutionary algorithm. In HS algorithm a bunch/group of solutions is randomly generated (Called Harmony memory). A new solution is generated by using all the solutions in the Harmony memory (rather than just two as used in GA) and if this new solution is better than the Worst solution in Harmony memory, the Worst solution gets replaced by this new solution. All though HS is a relatively new meta heuristic algorithm, its effectiveness and advantages have been demonstrated in various applications like design of municipal water distribution networks,[14] structural design,[15] traffic routing,[16] load dispatch problem in electrical engineering,[17] multi objective optimization,[18] rostering problems,[19] clustering,[20] classification and feature selection [21][22] to name a few. A detailed survey on applications of HS can be found in [23] and applications of HS in data mining can be found in.[24]
Artificial bee colony algorithm (Karaboga 2005)
Artificial bee colony algorithm is a meta-heuristic algorithm introduced by Karaboga in 2005,[25] and simulates the foraging behaviour of honey bees. The ABC algorithm has three phases: employed bee, onlooker bee and scout bee. In the employed bee and the onlooker bee phases, bees exploit the sources by local searches in the neighbourhood of the solutions selected based on deterministic selection in the employed bee phase and the probabilistic selection in the onlooker bee phase. In the scout bee phase which is an analogy of abandoning exhausted food sources in the foraging process, solutions that are not beneficial anymore for search progress are abandoned, and new solutions are inserted instead of them to explore new regions in the search space. The algorithm has a well-balanced exploration and exploitation ability.
Bees algorithm (Pham 2005)
The bees algorithm in its basic formulation was created by Pham and his co-workers in 2005,[26] and further refined in the following years.[27] Modelled on the foraging behaviour of honey bees, the algorithm combines global explorative search with local exploitative search. A small number of artificial bees (scouts) explores randomly the solution space (environment) for solutions of high fitness (highly profitable food sources), whilst the bulk of the population search (harvest) the neighbourhood of the fittest solutions looking for the fitness optimum. A deterministics recruitment procedure which simulates the waggle dance of biological bees is used to communicate the scouts' findings to the foragers, and distribute the foragers depending on the fitness of the neighbourhoods selected for local search. Once the search in the neighbourhood of a solution stagnates, the local fitness optimum is considered to be found, and the site is abandoned. In summary, the Bees Algorithm searches concurrently the most promising regions of the solution space, whilst continuously sampling it in search of new favourable regions.
Glowworm swarm optimization (Krishnanand & Ghose 2005)
The glowworm swarm optimization is a swarm intelligence optimization algorithm developed based on the behaviour of glowworms (also known as fireflies or lightning bugs). The GSO algorithm was developed and introduced by K.N. Krishnanand and Debasish Ghose in 2005 at the Guidance, Control, and Decision Systems Laboratory in the Department of Aerospace Engineering at the Indian Institute of Science, Bangalore, India.[28]
The behaviour pattern of glowworms which is used for this algorithm is the apparent capability of the glowworms to change the intensity of the luciferin emission and thus appear to glow at different intensities.
- The GSO algorithm makes the agents glow at intensities approximately proportional to the function value being optimized. It is assumed that glowworms of brighter intensities attract glowworms that have lower intensity.
- The second significant part of the algorithm incorporates a dynamic decision range by which the effect of distant glowworms are discounted when a glowworm has sufficient number of neighbours or the range goes beyond the range of perception of the glowworms.
The part 2 of the algorithm makes it different from other evolutionary multimodal optimization algorithms. It is this step that allows glowworm swarms to automatically subdivide into subgroups which can then converge to multiple local optima simultaneously, This property of the algorithm allows it to be used to identify multiple peaks of a multi-modal function and makes it a part of the evolutionary multimodal optimization algorithms family.
Shuffled frog leaping algorithm (Eusuff, Lansey & Pasha 2006)
The shuffled frog leaping algorithm is an optimization algorithm used in artificial intelligence.[29] It is comparable to a genetic algorithm.
Imperialist competitive algorithm (Atashpaz-Gargari & Lucas 2007)
The imperialist competitive algorithm is a computational method that is used to solve optimization problems of different types.[30] [31] Like most of the methods in the area of evolutionary computation, ICA does not need the gradient of the function in its optimization process. From a specific point of view, ICA can be thought of as the social counterpart of genetic algorithms (GAs). ICA is the mathematical model and the computer simulation of human social evolution, while GAs are based on the biological evolution of species.
This algorithm starts by generating a set of candidate random solutions in the search space of the optimization problem. The generated random points are called the initial Countries. Countries in this algorithm are the counterpart of Chromosomes in GAs and Particles in Particle Swarm Optimization (PSO) and it is an array of values of a candidate solution of optimization problem. The cost function of the optimization problem determines the power of each country. Based on their power, some of the best initial countries (the countries with the least cost function value), become Imperialists and start taking control of other countries (called colonies) and form the initial Empires.[30]
Two main operators of this algorithm are Assimilation and Revolution. Assimilation makes the colonies of each empire get closer to the imperialist state in the space of socio-political characteristics (optimization search space). Revolution brings about sudden random changes in the position of some of the countries in the search space. During assimilation and revolution a colony might reach a better position and has the chance to take the control of the entire empire and replace the current imperialist state of the empire.[32]
Imperialistic Competition is another part of this algorithm. All the empires try to win this game and take possession of colonies of other empires. In each step of the algorithm, based on their power, all the empires have a chance to take control of one or more of the colonies of the weakest empire.[30]
Algorithm continues with the mentioned steps (Assimilation, Revolution, Competition) until a stop condition is satisfied.
The above steps can be summarized as the below pseudocode.[31][32]
0) Define objective function:
1) Initialization of the algorithm. Generate some random solution in the search space and create initial empires.
2) Assimilation: Colonies move towards imperialist states in different in directions.
3) Revolution: Random changes occur in the characteristics of some countries.
4) Position exchange between a colony and Imperialist. A colony with a better position than the imperialist,
has the chance to take the control of empire by replacing the existing imperialist.
5) Imperialistic competition: All imperialists compete to take possession of colonies of each other.
6) Eliminate the powerless empires. Weak empires lose their power gradually and they will finally be eliminated.
7) If the stop condition is satisfied, stop, if not go to 2.
8) End
River formation dynamics (Rabanal, Rodríguez & Rubio 2007)
River formation dynamics is based on imitating how water forms rivers by eroding the ground and depositing sediments (the drops act as the swarm). After drops transform the landscape by increasing/decreasing the altitude of places, solutions are given in the form of paths of decreasing altitudes. Decreasing gradients are constructed, and these gradients are followed by subsequent drops to compose new gradients and reinforce the best ones. This heuristic optimization method was first presented in 2007 by Rabanal et al.[33] The applicability of RFD to other NP-complete problems has been studied,[34] and the algorithm has been applied to fields such as routing[35] and robot navigation.[36]
Intelligent water drops algorithm (Shah-Hosseini 2007)
Intelligent water drops algorithm contains a few essential elements of natural water drops and actions and reactions that occur between river's bed and the water drops that flow within. The IWD was first introduced for the traveling salesman problem in 2007.[37]
Almost every IWD algorithm is composed of two parts: a graph that plays the role of distributed memory on which soils of different edges are preserved, and the moving part of the IWD algorithm, which is a few number of Intelligent water drops. These intelligent water drops (IWDs) both compete and cooperate to find better solutions and by changing soils of the graph, the paths to better solutions become more reachable. It is mentioned that the IWD-based algorithms need at least two IWDs to work.
The IWD algorithm has two types of parameters: static and dynamic parameters. Static parameters are constant during the process of the IWD algorithm. Dynamic parameters are reinitialized after each iteration of the IWD algorithm. The pseudo-code of an IWD-based algorithm may be specified in eight steps:
- 1) Static parameter initialization
- a) Problem representation in the form of a graph
- b) Setting values for static parameters
- 2) Dynamic parameter initialization: soil and velocity of IWDs
- 3) Distribution of IWDs on the problem’s graph
- 4) Solution construction by IWDs along with soil and velocity updating
- a) Local soil updating on the graph
- b) Soil and velocity updating on the IWDs
- 5) Local search over each IWD’s solution (optional)
- 6) Global soil updating
- 7) Total-best solution updating
- 8) Go to step 2 unless termination condition is satisfied
Gravitational search algorithm (Rashedi, Nezamabadi-pour & Saryazdi 2009)
Gravitational search algorithm based on the law of gravity and the notion of mass interactions. The GSA algorithm uses the theory of Newtonian physics and its searcher agents are the collection of masses. In GSA, there is an isolated system of masses. Using the gravitational force, every mass in the system can see the situation of other masses. The gravitational force is therefore a way of transferring information between different masses (Rashedi, Nezamabadi-pour and Saryazdi 2009).[38] In GSA, agents are considered as objects and their performance is measured by their masses. All these objects attract each other by a gravity force, and this force causes a movement of all objects globally towards the objects with heavier masses. The heavy masses correspond to good solutions of the problem. The position of the agent corresponds to a solution of the problem, and its mass is determined using a fitness function. By lapse of time, masses are attracted by the heaviest mass, which would ideally present an optimum solution in the search space. The GSA could be considered as an isolated system of masses. It is like a small artificial world of masses obeying the Newtonian laws of gravitation and motion (Rashedi, Nezamabadi-pour and Saryazdi 2009). A multi-objective variant of GSA, called MOGSA, was first proposed by Hassanzadeh et al. in 2010.[39]
Cuckoo search (Yang & Deb 2009)
In operations research, cuckoo search is an optimization algorithm developed by Xin-she Yang and Suash Deb in 2009.[40][41] It was inspired by the obligate brood parasitism of some cuckoo species by laying their eggs in the nests of other host birds (of other species). Some host birds can engage direct conflict with the intruding cuckoos. For example, if a host bird discovers the eggs are not their own, it will either throw these alien eggs away or simply abandon its nest and build a new nest elsewhere. Some cuckoo species such as the New World brood-parasitic Tapera have evolved in such a way that female parasitic cuckoos are often very specialized in the mimicry in colors and pattern of the eggs of a few chosen host species [42]
Bat algorithm (Yang 2010)
Bat algorithm is a swarm-intelligence-based algorithm, inspired by the echolocation behavior of microbats. BA automatically balances exploration (long-range jumps around the global search space to avoid getting stuck around one local maximum) with exploitation (searching in more detail around known good solutions to find local maxima) by controlling loudness and pulse emission rates of simulated bats in the multi-dimensional search space.[43]
Flower pollination algorithm (Yang 2012)
Flower pollination algorithm is a metaheuristic algorithm that was developed by Xin-She Yang,[44] based on the pollination process of flowering plants.
This algorithm has 4 rules or assumptions:
- Biotic and cross-pollination is considered as global pollination process with pollen carrying pollinators performing Levy flights.
- Abiotic and self-pollination are considered as local pollination.
- Flower constancy can be considered as the reproduction probability is proportional to the similarity of two flowers involved.
- Local pollination and global pollination is controlled by a switch probability . Due to the physical proximity and other factors such as wind, local pollination can
have a significant fraction p in the overall pollination activities.
These rules can be translated into the following updating equations:
where is the solution vector and is the current best found so far during iteration. The switch probability between two equations during iterations is . In addition, is a random number drawn from a uniform distribution. is a step size drawn from a Lévy distribution.
Lévy flights using Lévy steps is a powerful random walk because both global and local search capabilities can be carried out at the same time. In contrast with standard Random walks, Lévy flights have occasional long jumps, which enable the algorithm to jump out any local valleys. Lévy steps obey the following approximation:
where is the Lévy exponent.[45] It may be challenging to draw Lévy steps properly, and a simple way of generating Lévy flights is to use two normal distributions and by a transform[46]
with
where is a function of .
Cuttlefish optimization algorithm (Eesa, Mohsin, Brifcani & Orman 2013)
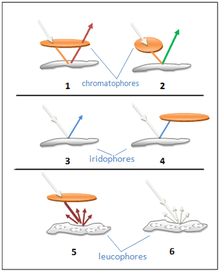
The cuttlefish optimization algorithm is a population-based search algorithm inspired by skin color changing behaviour of Cuttlefish which was developed in 2013 [47][48] It has two global search and two local search.
The algorithm considers two main processes: Reflection and Visibility. Reflection process simulates the light reflection mechanism, while visibility simulates the visibility of matching patterns. These two processes are used as a search strategy to find the global optimal solution. The formulation of finding the new solution (newP) by using reflection and visibility is as follows:
CFA divide the pubulaion to 4 Groups (G1, G2, G3 and G4). For G1 the algorithm appling case 1 and 2 (the interaction between chromatophores and iridophores) to produce a new solutions. These two cases are used as a golobal search. For G2, the algorithm uses case 3 (Iridophores reflection opaerator) and case 4 (the interaction between Iridophores and chromatophores) to produces a new solutions) as a local search. While for G3 the interaction between the leucophores and chromatophores (case 5) is used to produse solutions around the best solution (local search). Finaly for G4, case 6 (reflection operator of leucophores) is used as a global search by reflecting any incoming light as it with out any modification. The main step of CFA is described as follows:
1 Initialize population (P[N]) with random solutions, Assign the values of r1, r2, v1, v2.
2 Evaluate the population and Keep the best solution.
3 Divide population into four groups (G1, G2, G3 and G4).
4 Repeat
4.1 Calculate the average value of the best solution.
4.2 for (each element in G1)
generate new solution using Case(1 and 2)
4.3 for (each element in G2)
generate new solution using Case(3 and 4)
4.4 for (each element in G3)
generate new solution using Case(5)
4.5 for (each element in G4)
generate new solution using Case(6)
4.6 Evaluate the new solutions
5. Until (stopping criterion is met)
6. Return the best solution
Equations that are used to calculate reflection and visibility for the four Groups are described bellow:
Case 1 and 2 for G1:
Case 3 and 4 for G2:
Case 5 for G3:
Case 6 for G4:
Where , are Group1 and Group2, i presents the element in G, j is the point of element in group G, Best is the best solution and presents the average value of the Best points. While R and V are two random numbers produced around zero such as between (-1, 1), R represents the degree of reflection, V represents the visibility degree of the final view of the pattern, upperLimit and lowerLimit are the upper limit and the lower limit of the problem domain.
Artificial swarm intelligence (Rosenberg 2014)
Artificial swarm intelligence refers to a real-time closed-loop system of human users connected over the internet and structured in a framework modeled after natural swarms such that it evokes the group's collective wisdom as a unified emergent intelligence.[49][50] In this way, human swarms can answer questions, make predictions, reach decisions, and solve problems by collectively exploring a diverse set of options and converging on preferred solutions in synchrony. Invented by Dr. Louis Rosenberg in 2014, the ASI methodology has become notable for its ability to make accurate collective predictions that outperform the individual members of the swarm.[51] In 2016 an Artificial Swarm Intelligence from Unanimous A.I. was challenged by a reporter to predict the winners of the Kentucky Derby, and successfully picked the first four horses, in order, beating 540 to 1 odds.[52][53]
Criticism of the metaphor methodology
While individual metaphor-inspired metaheuristics have produced remarkably effective solutions to specific problems,[54] metaphor-inspired metaheuristics in general have attracted criticism in the research community for hiding their lack of effectiveness or novelty behind an elaborate metaphor.[54][55] Kenneth Sörensen noted that:[56]
In recent years, the field of combinatorial optimization has witnessed a true tsunami of "novel" metaheuristic methods, most of them based on a metaphor of some natural or man-made process. The behavior of virtually any species of insects, the flow of water, musicians playing together – it seems that no idea is too far-fetched to serve as inspiration to launch yet another metaheuristic. [I] will argue that this line of research is threatening to lead the area of metaheuristics away from scientific rigor.
Sörensen and Glover stated that:[57]
A large (and increasing) number of publications focuses on the development of (supposedly) new metaheuristic frameworks based on metaphors. The list of natural or man-made processes that has been used as the basis for a metaheuristic framework now includes such diverse processes as bacterial foraging, river formation, biogeography, musicians playing together, electromagnetism, gravity, colonization by an empire, mine blasts, league championships, clouds, and so forth. An important subcategory is found in metaheuristics based on animal behavior. Ants, bees, bats, wolves, cats, fireflies, eagles, dolphins, frogs, salmon, vultures, termites, flies, and many others, have all been used to inspire a "novel" metaheuristic. [...] As a general rule, publication of papers on metaphor-based metaheuristics has been limited to second-tier journals and conferences, but some recent exceptions to this rule can be found. Sörensen (2013) states that research in this direction is fundamentally flawed. Most importantly, the author contends that the novelty of the underlying metaphor does not automatically render the resulting framework "novel". On the contrary, there is increasing evidence that very few of the metaphor-based methods are new in any interesting sense.
In response, Springer's Journal of Heuristics has updated their editorial policy to state that:[58]
Proposing new paradigms is only acceptable if they contain innovative basic ideas, such as those that are embedded in classical frameworks like genetic algorithms, tabu search, and simulated annealing. The Journal of Heuristics avoids the publication of articles that repackage and embed old ideas in methods that are claimed to be based on metaphors of natural or manmade systems and processes. These so-called "novel" methods employ analogies that range from intelligent water drops, musicians playing jazz, imperialist societies, leapfrogs, kangaroos, all types of swarms and insects and even mine blast processes (Sörensen, 2013). If a researcher uses a metaphor to stimulate his or her own ideas about a new method, the method must nevertheless be translated into metaphor-free language, so that the strategies employed can be clearly understood, and their novelty is made clearly visible. (See items 2 and 3 below.) Metaphors are cheap and easy to come by. Their use to "window dress" a method is not acceptable."
[...] Implementations should be explained by employing standard optimization terminology, where a solution is called a "solution" and not something else related to some obscure metaphor (e.g., harmony, flies, bats, countries, etc.).
[...] The Journal of Heuristics fully endorses Sörensen’s view that metaphor-based “novel” methods should not be published if they cannot demonstrate a contribution to their field. Renaming existing concepts does not count as a contribution. Even though these methods are often called “novel”, many present no new ideas, except for the occasional marginal variant of an already existing methodology. These methods should not take the journal space of truly innovative ideas and research. Since they do not use the standard optimization vocabulary, they are unnecessarily difficult to understand.
Notes
- ↑ A. Colorni, M. Dorigo et V. Maniezzo, Distributed Optimization by Ant Colonies, actes de la première conférence européenne sur la vie artificielle, Paris, France, Elsevier Publishing, 134-142, 1991.
- ↑ M. Dorigo, Optimization, Learning and Natural Algorithms, PhD thesis, Politecnico di Milano, Italy, 1992.
- ↑ Zlochin, Mark; Birattari, Mauro; Meuleau, Nicolas; Dorigo, Marco (1 October 2004). "Model-Based Search for Combinatorial Optimization: A Critical Survey". Annals of Operations Research. 131 (1-4): 373–395. doi:10.1023/B:ANOR.0000039526.52305.af. ISSN 0254-5330.
- ↑ Kennedy, J.; Eberhart, R. (1995). "Particle Swarm Optimization". Proceedings of IEEE International Conference on Neural Networks. IV. pp. 1942–1948. doi:10.1109/ICNN.1995.488968.
- ↑ Shi, Y.; Eberhart, R.C. (1998). "A modified particle swarm optimizer". Proceedings of IEEE International Conference on Evolutionary Computation. pp. 69–73.
- ↑ Kennedy, J. (1997). "The particle swarm: social adaptation of knowledge". Proceedings of IEEE International Conference on Evolutionary Computation. pp. 303–308.
- ↑ Kennedy, J.; Eberhart, R.C. (2001). Swarm Intelligence. Morgan Kaufmann. ISBN 1-55860-595-9.
- ↑ Poli, R. (2007). "An analysis of publications on particle swarm optimisation applications" (PDF). Technical Report CSM-469. Department of Computer Science, University of Essex, UK.
- ↑ Poli, R. (2008). "Analysis of the publications on the applications of particle swarm optimisation" (PDF). Journal of Artificial Evolution and Applications. 2008: 1–10. doi:10.1155/2008/685175.
- ↑ Bonyadi, M. R.; Michalewicz, Z. (2016). "Particle swarm optimization for single objective continuous space problems: a review". Evolutionary Computation. in press.
- ↑ Geem, Zong Woo, Joong Hoon Kim, and G. V. Loganathan (2001). "A new heuristic optimization algorithm: harmony search." Simulation 76.2: pp. 60-68.
- ↑ Dennis Weyland (2015). "A critical analysis of the harmony search algorithm—How not to solve sudoku". Operations Research Perspectives.
- ↑ "Saka,M,P.,Hasancebi,O.,2009. Adaptive harmony search algorithm for design code optimization of steel structures,in: Harmony Search Algorithms for Structural Design Optimization.". . Springer, pp. 79–120.
- ↑ "Geem, Z.W., 2006. Optimal cost design of water distribution networks using harmony search". . Engineering Optimization 38, 259–277.
- ↑ "Gholizadeh, S., Barzegar, A., 2013. Shape optimization of structures for frequency constraints by sequential harmony search algorithm". Engineering Optimization 45, 627–646.
- ↑ "Geem, Z.W., Lee, K.S., Park, Y., 2005. Application of harmony search to vehicle routing". American Journal of Applied Sciences 2, 1552–1557.
- ↑ "Wang, L., Li, L.p., 2013. An effective differential harmony search algorithm for the solving non-convex economic load dispatch problems". International Journal of Electrical Power & Energy Systems 44, 832–843.
- ↑ "Nekooei, K., Farsangi, M.M., Nezamabadi-Pour, H., Lee, K.Y., 2013. An improved multi-objective harmony search for optimal placement of dgs in distribution systems". . Smart Grid, IEEE Transactions on 4, 557–567.
- ↑ "Hadwan, M., Ayob, M., Sabar, N.R., Qu, R., 2013. A harmony search algorithm for nurse rostering problems.". Information Sciences 233, 126–140.
- ↑ "Hoang, D.C., Yadav, P., Kumar, R., Panda, S.K., 2014. Real-time implementation of a harmony search algorithm-based clustering protocol for energy-efficient wireless sensor networks". Industrial Informatics, IEEE Transactions on 10, 774–783.
- ↑ "]Diao, R., Shen, Q., 2012. Feature selection with harmony search.". Systems, Man, and Cybernetics, Part B: Cybernetics, IEEE Transactions on 42, 1509–1523.
- ↑ "Fattahi, H., Gholami, A., Amiribakhtiar, M.S., Moradi, S., 2015. Estimation of asphaltene precipitation from titration data: a hybrid support vector regression with harmony search". Neural Computing and Applications 26, 789–798.
- ↑ "Manjarres, D., Landa-Torres, I., Gil-Lopez, S., Del Ser, J., Bilbao, M.N., Salcedo-Sanz, S., Geem, Z.W., 2013. A survey on applications of the harmony search algorithm". Engineering Applications of Artificial Intelligence 26, 1818–1831.
- ↑ "Assad,A.,Deep,K.,2016. Applications of harmony search algorithm in data mining: A survey". Proceedings of Fifth International Conference on Soft Computing for Problem Solving, Springer. pp. 863–874.
- ↑ Karaboga, Dervis (2010). "Artificial bee colony algorithm". Scholarpedia. 5 (3): 6915. doi:10.4249/scholarpedia.6915.
- ↑ Pham DT, Ghanbarzadeh A, Koc E, Otri S, Rahim S and Zaidi M. The Bees Algorithm. Technical Note, Manufacturing Engineering Centre, Cardiff University, UK, 2005.
- ↑ Pham, D.T.; Castellani, M. (2009). "The Bees Algorithm – Modelling Foraging Behaviour to Solve Continuous Optimisation Problems". Proc. ImechE, Part C. 223 (12): 2919–2938. doi:10.1243/09544062jmes1494.
- ↑ Krishnanand K.N. and D. Ghose (2005) "Detection of multiple source locations using a glowworm metaphor with applications to collective robotics". IEEE Swarm Intelligence Symposium, Pasadena, California, USA, pp. 84–91.
- ↑ Muzaffar Eusuff , Kevin Lansey & Fayzul Pasha (2006) Shuffled frog-leaping algorithm: a memetic meta-heuristic for discrete optimization, Engineering Optimization, 38:2, 129–154, DOI: 10.1080/03052150500384759
- 1 2 3 Atashpaz-Gargari, E.; Lucas, C (2007). "Imperialist Competitive Algorithm: An algorithm for optimization inspired by imperialistic competition". IEEE Congress on Evolutionary Computation. 7. pp. 4661–4666.
- 1 2 Hosseini, S.; Al Khaled, A. (2014). "A survey on the Imperialist Competitive Algorithm metaheuristic: Implementation in engineering domain and directions for future research". Applied Soft Computing. 24: 1078–1094.
- 1 2 Nazari-Shirkouhi, S.; Eivazy, H.; Ghodsi, R.; Rezaie, K.; Atashpaz-Gargari, E. (2010). "Solving the Integrated Product Mix-Outsourcing Problem by a Novel Meta-Heuristic Algorithm: Imperialist Competitive Algorithm". Expert Systems with Applications. 37 (12): 7615–7626. doi:10.1016/j.eswa.2010.04.081.
- ↑ P. Rabanal, I. Rodríguez, and F. Rubio (2007) "Using River Formation Dynamics to Design Heuristic Algorithms". Unconventional Computation, Springer, LNCS 4616, pp. 163–177.
- ↑ P. Rabanal, I. Rodríguez, and F. Rubio (2009) "Applying River Formation Dynamics to Solve NP-Complete Problems". Nature-Inspired Algorithms for Optimisation, Springer, SCI 193, pp. 333–368.
- ↑ S.H. Amin, H.S. Al-Raweshidy, R.S. Abbas (2014) "Smart data packet ad hoc routing protocol", Computer Networks, Elsevier, Volume 62, pp. 162-181
- ↑ G. Redlarski, A. Pałkowski, M. Dąbkowski (2013), "Using River Formation Dynamics Algorithm in Mobile Robot Navigation", Solid State Phenomena, Volume 198, pp. 138-143
- ↑ Shah-Hosseini, H. (2009). "The intelligent water drops algorithm: a nature-inspired swarm-based optimization algorithm". International Journal of Bio-Inspired Computation. 1 (1/2): 71–79. doi:10.1504/ijbic.2009.022775.
- ↑ Rashedi, E.; Nezamabadi-pour, H.; Saryazdi, S. (2009). "GSA: a gravitational search algorithm". Information Science. 179 (13): 2232–2248. doi:10.1016/j.ins.2009.03.004.
- ↑ Hassanzadeh, Hamid Reza, and Modjtaba Rouhani. "A multi-objective gravitational search algorithm." Computational Intelligence, Communication Systems and Networks (CICSyN), 2010 Second International Conference on. IEEE, 2010.
- ↑ X.-S. Yang; S. Deb (December 2009). Cuckoo search via Lévy flights. World Congress on Nature & Biologically Inspired Computing (NaBIC 2009). IEEE Publications. pp. 210–214. arXiv:1003.1594v1
.
- ↑ Inderscience (27 May 2010). "Cuckoo designs spring". Alphagalileo.org. Retrieved 2010-05-27.
- ↑ R. B. Payne, M. D. Sorenson, and K. Klitz, The Cuckoos, Oxford University Press, (2005).
- ↑ X. S. Yang, A New Metaheuristic Bat-Inspired Algorithm, in: Nature Inspired Cooperative Strategies for Optimization (NISCO 2010) (Eds. J. R. Gonzalez et al.), Studies in Computational Intelligence, Springer Berlin, 284, Springer, 65-74 (2010).
- ↑ Xin-She Yang. Flower pollination algorithm for global optimization, Unconventional Computation and Natural Computation. Lecture Notes in Computer Science. Volume 7445, pp. 240-249 (2012).
- ↑ I. Pavlyukevich, Lévy flights, non-local search and simulated annealing, J. Computational Physics, Vol. 226, 1830-1844 (2007).
- ↑ X. S. Yang, Nature-Inspired Optimization Algorithms, Elsevier, (2014).
- ↑ Adel Sabry Eesa, A. M. A. B., Zeynep Orman. (2013). Cuttlefish Algorithm – A Novel Bio-Inspired Optimization Algorithm. International Journal of Scientific & Engineering Research, 4(9).
- ↑ Adel Sabry Eesa, Z. O., Adnan Mohsin Abdulazeez Brifcani. (2015). A novel feature-selection approach based on the cuttlefish optimization algorithm for intrusion detection systems. Expert Systems with Applications, 42, 2670–2679. doi: 10.1016/j.eswa.2014.11.009.
- ↑ Rosenberg, Louis (February 12, 2016). "Artificial Swarm Intelligence, a Human-in-the-loop approach to A.I.". Proceedings of the 13th Annual AAAI Conference on Artificial Intelligence (AAAI-16).
- ↑ Reese, Hope (Jan 22, 2016). "How 'artificial swarm intelligence' uses people to make better predictions than experts".
- ↑ Rosenberg, Louis (2015). "Human swarming, a real-time method for parallel distributed intelligence". Swarm/Human Blended Intelligence (SHBI), 2015. doi:10.1109/SHBI.2015.7321685.
- ↑ CUTHBERTSON, ANTHONY (May 10, 2016). "ARTIFICIAL INTELLIGENCE TURNS $20 INTO $11,000 IN KENTUCKY DERBY BET (Newsweek)".
- ↑ Ohlheiser, Abby (June 2, 2016). "What happened when an A.I. hive mind answered Reddit's burning politics questions (Washington Post)".
- 1 2 Alexander Brownlee and John R. Woodward (2015). "Why we fell out of love with algorithms inspired by nature". The Conversation.
- ↑ Jerry Swan, Steven Adriaensen, Mohamed Bishr, Edmund K. Burke, John A. Clark, Patrick De Causmaecker, Juanjo Durillo, Kevin Hammond, Emma Hart, Colin G. Johnson, Zoltan A. Kocsis, Ben Kovitz, Krzysztof Krawiec, Simon Martin, J. J. Merelo, Leandro L. Minku, Ender Özcan, Gisele L. Pappa, Erwin Pesch, Pablo Garcáa-Sánchez, Andrea Schaerf, Kevin Sim, Jim E. Smith, Thomas Stützle, Stefan Voß, Stefan Wagner, Xin Yao. "A Research Agenda for Metaheuristic Standardization". "Metaphors often inspire new metaheuristics, but without mathematical rigor, it can be hard to tell if a new metaheuristic is really distinct from a familiar one. For example, mathematically, 'Harmony search' turned out to be a simple variant of 'Evolution Strategies' even though the metaphors that inspired them were quite different. Formally describing state, representation, and operators allows genuine novelty to be distinguished from minor variation."
- ↑ Sörensen, Kenneth (2013). "Metaheuristics—the metaphor exposed". International Transactions in Operational Research. 22: 3–18. doi:10.1111/itor.12001.
- ↑ Fred Glover and Kenneth Sörensen (ed.). "Metaheuristics". Scholarpedia.
- ↑ Journal of Heuristic Policies on Heuristic Search Research. Springer.
References
- Sörensen, Kenneth; Sevaux, Marc; Glover, Fred. "A History of Metaheuristics" (PDF). In Martí, Rafael; Panos, Pardalos; Resende, Mauricio. Handbook of Heuristics. Springer. ISBN 978-3-319-07123-7.
- Sörensen, Kenneth (2013). "Metaheuristics—the metaphor exposed". International Transactions in Operational Research. 22: 3–18. doi:10.1111/itor.12001.
- Lones, Michael A. (2014). "Metaheuristics in Nature-Inspired Algorithms" (PDF). GECCO '14. doi:10.1145/2598394.2609841.
- Fister Jr., Iztok; Yang, Xin-She; Fister, Iztok; Brest, Janez; Fister, Dušan (2013). "A Brief Review of Nature-Inspired Algorithms for Optimization". arXiv:1307.4186
[cs.NE].
See also
External links
- Evolutionary Computation Bestiary — a tongue-in-cheek account of all the weird, even bizarre metaphor-based metaheuristics out there in the wide world of academic publishing